DataTribe Insights Q3 2023: Powering Forward – Even with the 50lb Fed Backpack On
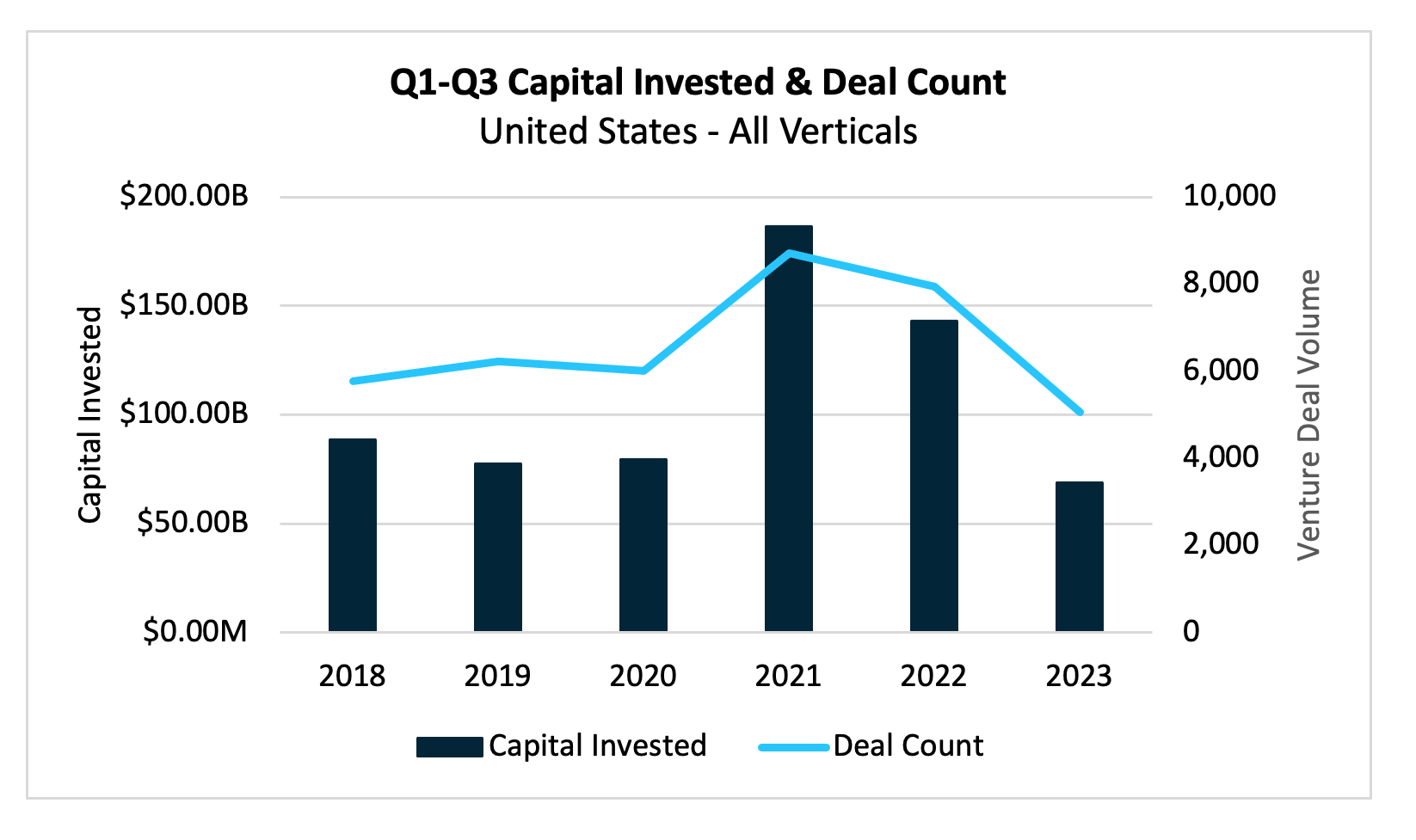
1. Q3 Cybersecurity Investment Uptick
A total of $1.2 billion was invested across 70 cyber deals, marking an uptick from $790 million across 57 deals in Q2. This rebound has been most notable in the seed and Series A funding stages, which saw increases of 67% and 37% in invested capital, respectively.
It was a busy quarter in the world of cyber investment. Funding activity for early-stage and Series A companies rose significantly. At DataTribe, we invested $2 million in Fianu, a disruptor in automated software governance. Key findings and discussions from the Q3 2023 DataTribe Insights include:
2. Bigger Deals, Smaller Numbers
Late-stage growth capital (Series C and beyond) within the broader market, specifically cybersecurity, has continued to trail behind previous years. Year-to-date through Q3, the deal volume has decreased significantly by 75%, dropping from 44 deals last year to just 14 in the current year.
3. Only as Strong as the Weakest Component
The 2023 DataTribe Challenge highlighted an increased focus on securing the building blocks of hardware and software products. Leveraging the value of new data sets to include hardware and software bill of materials (HBOMs and SBOMs) is an over-the-horizon opportunity to meet the challenges posed by an increased focus on software and hardware supply chain security.
4. Moving Beyond Passwords, What’s Next?
Before we can bury passwords forever, we need a plausible alternative that combines usability and security. What is a passkey, and why is it necessary for a password-less login? How does it differ from a traditional password, and is it more effective than Multi-Factor Authentication (MFA)? Are there superior alternatives to a passkey? We take a look.
5. AI Risk Management
AI offers tremendous promise but also brings some significant risks. It will be necessary to ensure that the rewards far outweigh the risks. Managing AI’s risks requires a blend of technical, legal, and ethical perspectives, examining AI through real-world applications, bias detection, and transparency in decision-making.
Q3 2023 provided some additional domestic economic and regulatory clarity, while geopolitical issues led by conflict in the Middle East are tenuous, to put it lightly.
The Federal Reserve has indicated higher interest rates for the long term. The Biden Administration issued an executive order on artificial intelligence (AI) that holds promise for providing guardrails and guidance on the safe, secure, and innovative implementation of AI. But, the ramifications of the Hamas terrorist attack on Israel, resulting in Middle East conflict and nation-state adversary posturing, will be felt in Q4 and beyond.
In Q3, the economy continued to remain strong. GDP increased at a 4.9% annual rate, above estimates, and the unemployment rate remained steady at 3.8%, extending the longest stretch under 4% unemployment in almost 60 years. Wage growth remained steady – up 4.3% — and nonfarm productivity increased at an annualized rate of 4.7%, the fastest since the third quarter of 2020.
However, public equity markets reflected a lack of confidence in future growth. All major indices were below water in Q3, with the S&P 500 leading the way at a loss of over two percent. Yet, all significant markets remained in the black for the year.
Private markets continue to lag the 5-year average, with $73 billion invested across 5,200 deals through September. However, the cybersecurity sector continued to buck the headwinds and showed an increase in quarterly investment. A total of $1.2 billion was invested across 70 deals, increases of 34% and 22% over Q2.
But delving deeper into the numbers shows that rough sailing could still be ahead for Series B and larger companies whose capital runway may be ending. VC activity in cyber was highly focused on Seed and Series A funding of emerging companies, which saw increases of 67% and 37% in invested capital, respectively.
In this quarter’s installment of DataTribe Insights, we dig into the latest venture market trends, discuss the innovations we unearthed during our annual DataTribe Challenge, highlight the potential death of the password, and a Q3 Quarterly Insights would not be complete without a look at AI.
In the third quarter of 2023, the wave of public market optimism that buoyed the first half of the year began to recede. The Federal Reserve’s signal that interest rates would remain ‘higher for longer,’ along with mounting geopolitical turmoil, suggest that public markets will face continued challenges as we move into Q4, a quarter that has historically boasted the highest average returns. This pervasive macroeconomic uncertainty has rippled through the investment ecosystem, affecting limited partners, venture funds, and private companies.
In analyzing data from PitchBook, through Q3, the overall deal and capital invested in U.S. private markets has continued to lag the prior five years, with $73 billion invested year to date across approximately 5,200 deals. The downturn in activity—potentially due to the retreat of non-traditional investors, the interest rates environment, and a challenging exit landscape—may signal a rough journey ahead, particularly for companies nearing the end of their cash runway from the robust deal activity seen in 2021-2022.
Amidst this contraction, the cybersecurity sector has remained resilient, with an overall quarterly increase in investment activity. A total of $1.2 billion was invested across 70 deals, marking an uptick from $790 million across 57 deals in Q2. This rebound has been most notable in the seed and Series A funding stages, which saw increases of 67% and 37% in invested capital, respectively.
During the quarter, 46 seed rounds were completed, reflecting continued interest in early-stage cybersecurity ventures despite the climate of caution. The median deal size slightly increased to $4.0 million from $3.9 million. Median pre-money valuations at the seed stage grew by 26% quarter over quarter, reaching $17.0 million—a figure surpassing the high of $15.8 million recorded in Q4 2022. The range of pre-money valuations stretched from $3.6 to $45.0 million, with the top end of the range dominated by companies leveraging generative AI/ML for cybersecurity.
Series A deals increased by one quarter-over-quarter to fifteen in Q3. Investors are still displaying a general flight to quality, and as discussed in previous reports, the performance bar that will attract venture capital remains exceptionally high. Like the seed stage, we see a wide dispersion in pre-money valuations spanning $16 million to $475 million, with top-end valuations centered around generative AI/ML and Web3 security technologies.
Series B rounds have continued to stall, with only two deals completed during Q3, which is consistent with the previous quarter.
Late-stage growth capital (Series C and beyond) within the broader market, specifically cybersecurity, has continued to trail behind previous years. During Q3, there were six growth-stage cybersecurity deals compared to four in the prior quarter. Year-to-date through Q3, the deal volume has decreased significantly by 75%, dropping from 44 deals last year to just 14 in the current year. Despite venture capitalists having record levels of dry powder, there has been a noticeable shift in capital deployment. Emphasis on supporting existing portfolio companies and a tighter focus on company fundamentals are tempering the cadence of new deals, particularly at growth stages.
While the next couple of quarters may be tough as the macroeconomic factors play out, especially for current companies heading to their next financing round, it’s a moment to separate the cream, milk, and curd and focus venture dollars on capitalizing the truly differentiated opportunities. We remain optimistic that the winners in this market will be transformational companies leading the charge into the next evolution of cybersecurity.
One of the major highlights of Q3 for us was the DataTribe Challenge. Technically, the Challenge Finalists event took place in Q4, but all the lead-up to it was in Q3.
First, thank you to all the founding teams that applied to the Challenge. We received a record number of submissions this year, and the quality was incredibly high. We never cease to be amazed and inspired by the innovativeness and entrepreneurial spirit of the teams that apply to the Challenge. It was not easy for us to narrow down to the five Finalists we had this year: Vigilant Ops, LeakSignal, Dapple, Ampsight, and Ceritas.
One major observation from the Challenge this year is the ascendency of AI in this post-ChatGPT year. At this time in 2022, we saw a significant number of Challenge applications that were centered on Web3-related security. This year, the focus in Web3 has been almost completely replaced by founders focusing on applying AI to security problems. In general, where there is data, there are founders working on cybersecurity products leveraging AI. Examples include using AI to AppSec scans, PCAP data, security alerts, and malware. The goals are to make security less labor intensive, tease out subtle patterns humans may miss, reduce response times, and predict threats to get ahead of adversaries. In addition, companies are also addressing AI as a new attack surface, focusing on risks of data poisoning and overall governance (see the Insights piece later in this report about AI Risk Management).
Another interesting observation from the Challenge this year is the increasing desire for customers to understand the security posture of components that make up end products — software and hardware. Incidents like Log4j elevated the collective consciousness among security practitioners that vulnerabilities lie below the surface of products and systems. With regulatory pressure spurring adoption, we are seeing this industry-wide realization now play out in founders working to bring to market products that help automate processes around software and hardware supply chain security and leverage the value of new data sets such as SBOMs.
As is typically the case with the Challenge, the Finalists tend to each exemplify interesting trends. Here’s a rundown:
Vigilant Ops (Overall Winner):
https://www.vigilant-ops.com/
Trend: Software Supply Chain Security and the Push for SBOMs.
What They Do: Vigilant Ops, the overall Challenge Winner, provides a complete SBOM management platform and clearinghouse — for both software producers and buyers.
LeakSignal:
https://www.leaksignal.com/
Trend: The need to adapt security tooling to accommodate the new requirements of modern microservice architectures.
What They Do: High-performance, easy-to-install microservice security service that can inspect traffic flowing the microservice-based infrastructure in real-time and enforce filtering and blocking rules in-line.
Dapple Security:
https://dapplesecurity.com/
Trend: The need for easier, more secure identity and access management. In addition, the need to reduce the security risk associated with the proliferation of personally identifiable information through data breaches.
What They Do: Dapple Security provides customers the ability to securely log into systems without storing sensitive identity data. The secret data needed to authenticate is regenerated on the fly at the time of login. And since there is no need to store sensitive user data, Dapple Security prevents phishing and related attacks that rely on stolen credentials, preserving user privacy and dramatically reducing the data attack surface.
Ampsight:
https://www.ampsight.com/
Trend: AI/ML models are highly opaque, especially to people outside of data science teams. Increasingly, business stakeholders and regulators will want (and need) to have governance controls and visibility into the AI models used in their business.
What They Do: Designed for CISOs, Nucleus is an AI Risk Management solution that automatically generates risk and trustworthiness scorecards and recommendations that ensure AI/ML models are properly used within an organization.
Ceritas:
https://ceritas.ai/
Trend: The need for comprehensive visibility into what components and sub-components make up hardware products to comply with banned product lists and manage hardware vulnerabilities.
What They Do: Ceritas provides Hardware Bill of Materials (HBOM) vulnerability analysis and Digital Bill of Materials (DBOM) data transfer for customers.
With submissions from all over the world, from the overall vantage of the DataTribe Challenge, seed-stage innovation in cybersecurity is as vibrant as ever.
By now, you’ve probably come across the term “Passkey.” Maybe somebody used the term when describing this magical thing called “password-less.” Or perhaps you received a message in the last month or two from Google or Apple asking you to setup a passkey and use it to log in to their services. This may have sparked some questions for you.
What is a passkey, and why is it necessary for a password-less login? How does it differ from a traditional password, and is it more effective than Multi-Factor Authentication (MFA)? Are there superior alternatives to a passkey? Here’s a brief overview of the future of authentication.
Passkeys are private keys securely stored on a local device, enabling login without passing around a password, hence facilitating ‘password-less’ login. They operate based on the FIDO2 standard, which, although existing for the past 7-8 years, is now actively promoted by major players like Google and Apple as the preferred method for logging into their services. As a result, they are likely to become increasingly common. For instance, in September, Microsoft announced an update to Windows 11 to enhance passkey support. Following this, Google recently released Credentials Manager, a library allowing developers to implement passkey usage in their Android apps easily. Moreover, just a few weeks ago, Google began prompting users to set up passkeys on their accounts (such as Gmail and Google Workspace accounts).
The concept behind passkeys is they don’t involve sending around sensitive information during authentication, making them relatively more secure compared to passwords. In addition, passkeys are easier to use if you primarily access services through your personal devices (like a laptop, phone, or, for more advanced users, a hardware key). Once you verify your identity to the device, you can access all your services without the need to remember multiple passwords.
Passkeys also reduce the need for MFA. This is due to the significantly enhanced security they provide – being more difficult to hack or steal than passwords. That said, combining passkey with MFA technically offers an even higher level of security.
Despite all these benefits, passkey theft is possible. Furthermore, since these passkeys are stored on devices, they primarily authenticate the device rather than the user. This situation poses challenges when the device is lost, stolen, or replaced with a newer model. Losing access to the device means losing the ability to authenticate, potentially allowing anyone with access to the device to log in using that passkey.
So, are there innovations coming that go beyond passkeys? Yes.
During our recent DataTribe Challenge event, one of the finalists, Dapple Security, introduced a technology that doesn’t store the passkey on a device. Instead, the passkey is dynamically generated, whenever needed, using biometric data. This isn’t your father’s biometric login; no biometric data is stored for comparison. Instead, a biometric reading, known for its inherent variability and inability to produce the same data set, can consistently output the same private key every time, ensuring its reliability. When a passkey is needed, a fingerprint or a face scan is used to generate it dynamically. It’s then used to sign an authentication message and immediately vanishes—never stored or transmitted anywhere. This method resolves the issue of traditional passkeys being stored on and linked to specific devices. With Dapple’s technology, passkeys are directly associated with actual humans. This heightened security approach eliminates concerns about device loss, allowing logins on any device as long as the biometrics are available.
Another technology expanding on the passkey concept is distributed keys, which are processed using Multi-Party Computation(MPC) technology. This has been more commonly applied in Web3 to safeguard wallet keys (similar to passkeys but with different standards). This approach involves dividing a key into separate pieces stored in different locations. As a result, it is very difficult for bad actors to steal the key. They would need to access multiple devices, each holding a segment of the key, to steal the entire key.
In addition, this approach allows for a built-in recovery process. For instance, signing algorithms necessary for authentication typically require some but not all of the private key segments to be merged into a complete key. If the key consists of five pieces and the algorithm mandates three out of the five for authentication, the system can still authenticate even if one piece is lost by utilizing the remaining pieces.
These emerging innovations still require refinement and greater adoption before becoming mainstream. Nonetheless, they mark another stride toward more secure authentication and improved user experiences. In today’s zero-trust world, user identity IS the security perimeter. As a result, secure authentication is more essential than ever. All will undoubtedly welcome anything that streamlines this process.
This quarter, one of the DataTribe Challenge Finalists showed us an ambitious vision for the future of an interesting new category: AI Risk Management.
AI has evolved into a critical component, a stealthy yet ever-present power driving the smooth experiences that consumers and businesses now expect. AI isn’t just an add-on; it’s the foundation of a revolution in productivity, automating routine tasks and enabling remarkable achievements across various industries.
Yet, AI treads a fine line between risk and reward. Concerns about AI include ethical dilemmas and potential threats to our existence. Biases can creep into AI systems, reflecting and intensifying the prejudices in their training data, as seen in some facial recognition technologies that have shown lower accuracy for specific demographic groups. AI’s potential for surveillance poses risks to privacy and freedom if misused, highlighted by instances of state surveillance. Ethical questions also arise with autonomous weapons on the battlefield.
Real-world incidents have highlighted the dangers of AI, indicating its vulnerabilities and unintended outcomes. Autonomous vehicle mishaps have resulted in casualties, such as the Uber self-driving car incident in Arizona in 2018. A study in 2019 by NISTshowed that many facial recognition systems are less accurate for non-white and female faces, which can lead to injustices in law enforcement and hiring practices. The debacle with Microsoft’s AI chatbot “Tay,” which was manipulated into making offensive comments, showcased AI’s susceptibility to misuse and the need for protective measures. The 2010 flash crash, partly blamed on automated trading algorithms, revealed AI’s potential to trigger financial instability. More recently, generative AI has added complexity with concerns about disinformation, hyper-realistic phishing, and infringement on intellectual property rights with AI-generated content, to name a few.
The expanding field of AI risk assessment reflects our collective realization of AI’s promise and the need for scrutiny. Managing AI’s risks requires a blend of technical, legal, and ethical perspectives, examining AI through real-world applications, bias detection, and transparency in decision-making. As AI systems grow in complexity and influence, the risks of unintended effects increase. This necessitates a framework of regulation, ethical consideration, and inclusion of stakeholder input to align AI advancements with societal interests. This framework isn’t fixed and must evolve as AI does, ensuring innovation stays aligned with societal welfare.
Ignoring these risks can lead to individual harm, societal upheaval, and a loss of trust in AI. Some key concerns with machine learning (ML) include:
A burgeoning market for ML model risk assessment reflects the increased dependence on AI and the demand for accountability, fairness, and robustness. The market around assessing the risk with ML models will grow as AI becomes more integrated into critical decision-making processes, and stakeholders demand transparency and accountability. It’s a multidisciplinary field, combining expertise from machine learning, domain-specific knowledge, ethics, and regulatory compliance. This multidisciplinary market involves:
AmpSight, a finalist in the DataTribe Challenge, is an example of innovation in this field. Drawing on their experience with Project Maven, a Department of Defense computer vision project), AmpSight developed a risk-scoring system for ML models, featuring a unified dashboard that integrates proprietary and third-party tools. It assesses risks across multiple dimensions, ensuring AI systems are safe, reliable, understandable, secure, private, and unbiased.
The development of AI brings to mind a timeless truth: with great power comes immense responsibility. The future of AI will be shaped by the hands that steer it and the choices that direct it. Much faster than with other new categories (such as social media in the aughts), regulators and technologists are rapidly creating frameworks and tools to assist in measuring and governing AI risk.